Abandon compute thresholds at your peril
They are the best tool we have to reduce the burden of AI regulation
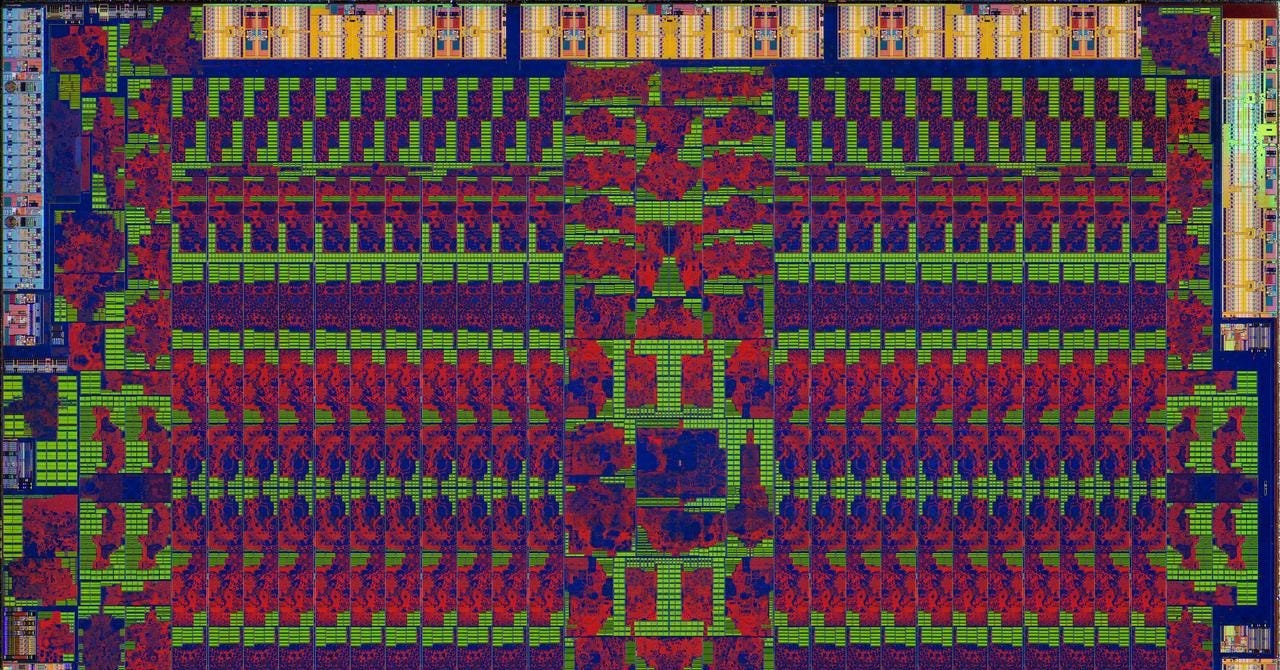
Compute thresholds have had a bumpy year. Last November they seemed on top of the world, taking centre-stage in the White House’s executive order on AI. The EU followed soon after. But more recently, they seem to be falling out of favour. Following the lead of certain academics, last month Gavin Newsom questioned their entire purpose. “Key to the debate,” he said, is “whether the threshold for regulation should be based on the cost and number of computations needed to develop an AI model, or whether we should evaluate the system’s actual risks regardless of these factors.” Some approvingly concurred.
But the backlash to training compute thresholds is ill-advised. As currently used, they are a sensible first step to identify models which deserve more regulatory scrutiny. A policy regime without them would be impractically onerous, punishing startups and serving only to entrench the market dominance of AI’s biggest companies — with only marginal benefits for risk reduction.
To understand why abandoning compute thresholds would be a mistake, we need to consider what they're intended to do. In the executive order and bills like SB 1047, they help governments identify and tackle a particular subset of risks, which we might call “anticipated” risks: risks arising from capabilities that AI models don’t have today, but that they may develop soon. Think of an AI model significantly improving an amateur’s attempt to build a bioweapon, or enabling catastrophic cyberattacks. Right now, such queries yield little more than what you'd get from Google. But as models improve, they may provide a meaningful marginal uplift to bad actors, significantly increasing the likelihood of catastrophe.
Regulators, understandably, would like to stop such things from happening. They are therefore asking companies to monitor their models for such capabilities. But what should be monitored?
The answer is simple: focus regulatory attention on the world's most advanced frontier models, and use compute thresholds to identify them. We know current models like GPT-4 can't design bioweapons. But such capabilities may emerge in future iterations. And while there’s debate about whether bigger always means better in AI — smaller models sometimes outperform larger ones — frontier language models have consistently been those trained with the most compute. Leading AI companies are doubling down on this scaling strategy, suggesting that these anticipated risks will likely first appear in models using unprecedented amounts of computing power. It would, therefore, be prudent to proactively test such models for these capabilities — and we can identify such models using compute thresholds.
As an example, we could set a threshold above GPT-4’s compute and below GPT-5’s anticipated compute. All models above that threshold should be tested for dangerous capabilities like biorisk, cyberrisk, and more speculative threats like self-replication. This is particularly practical given how frontier models tend to emerge in capability 'waves' — GPT-4, Claude 3.5, Gemini 1.0 and Llama 3.1 are all of roughly equivalent capabilities and were trained with similar compute. If we don’t see anything dangerous at the GPT-5 level, we can raise the threshold again.1 Hopefully, we’ll then catch these anticipated risks before they arise — and they can remain anticipated and unrealised.
Compute thresholds are not well-placed to tackle all risks: they are poorly suited to tackling many urgent problems, such as the proliferation of non-consensual sexual imagery or the racial bias of AI models, and other approaches are needed to address these harms. Nor should compute thresholds be the sole determinant of whether a model is allowed to be deployed. That would be foolish: compute is an imperfect proxy for risk, and there may be many large models which do not pose these anticipated risks. In line with the desire for ‘evidence-based policy’, rules for deployment should be based on whether the models actually pose a risk, which can only be determined by dangerous capability and sociotechnical evaluations.
But that does not entail abandoning compute thresholds altogether. When it comes to emerging risks, such thresholds can serve as an initial filter for which models to evaluate in the first place — as the Institute for Advanced Studies’ AI Policy and Governance Working Group has said, they can simply “help identify, delineate, and filter models”. The idea is a two-stage regulatory system: we monitor and test all models above a certain compute threshold for dangerous capabilities, but only impose restrictions on them based on the results of those tests.
The key advantage of this approach is its light-touch. Under this framework, we will not waste time or resources testing for dangerous capabilities in models which are very unlikely to have them. That lifts a significant burden on startups: with a compute threshold that slowly rises over time, the only companies who have to comply are a handful of the largest AI developers, such as OpenAI, Anthropic, and Google. Given the close correlation between compute and costs, it’s an excellent way to ensure pre-deployment testing is only forced on those who can afford it.
Critics of compute thresholds often fail to acknowledge an uncomfortable truth: their proposed alternatives would hurt AI startups. Such people argue, correctly, that compute thresholds are a crude and imperfect proxy for risk. But their solution — abandoning thresholds entirely, in favour of directly measuring a model’s dangerous capabilities — would require running expensive and time-consuming dangerous capability evaluations on every new AI model. Such a requirement would be a death knell for many startups, creating a regulatory burden that only the largest companies can afford to comply with. It would also be a waste of time: while it might catch some dangerous models that a compute-filtered approach would miss, it would do so at the expense of running thousands of evaluations on models that foreseeably do not pose a risk.
While we certainly need to evaluate models for dangerous capabilities before regulating them, we need some sort of threshold to decide which models to evaluate. Though other thresholds have been proposed, such as the number of users, the “demonstrated impact” of a model, or the amount of money spent on the model, these are even less correlated with model performance than training compute is. Worse, many can only be measured after deployment — too late to catch potential dangers.
Some worry that compute thresholds are not robust to the changing nature of AI development. They fear that decentralised training will make it much easier for actors to hide how much compute they’re using to train a model, allowing thresholds to be skirted. But the worry seems misplaced: given the cost of training a frontier model, training one surreptitiously is vanishingly unlikely. It’s hard to imagine a company that is willing to spend hundreds of millions developing a model and is also willing to brazenly lie to the government about it, with a good chance of them eventually getting caught.
Another concern is that models like o1 suggest we may be moving away from training compute as the primary determinant of capability. This means that compute thresholds may increasingly miss dangerous models that happen to fall under the set threshold.
But this is not an insurmountable challenge either. Instead of just considering training compute, we could also consider reinforcement learning, or even inference, compute. Or we could, as various versions of SB 1047 did, say that evaluations must be done on all models trained above a certain level of compute, with a certain amount of money, or on models with similar capabilities to those of the compute threshold. This is a bit of a fudge, particularly the latter clause. But in an industry which moves as fast as AI does, fudges are sometimes necessary: perfection is the enemy of the good.
Compute thresholds are not a silver bullet. But as an initial filter for regulatory scrutiny, they’re uniquely suited to catching anticipated risks before they materialise. Proposed alternatives either fail to address these risks or impose a much heavier regulatory burden. If we truly want to mitigate risks without stifling innovation, we should think hard before abandoning one of our most practical tools.
There are questions about whether, in the long-term, compute thresholds should be raised (so they only capture frontier models) or lowered (because algorithmic improvements will likely mean that dangerous capabilities will trickle down to smaller models). That’s a tricky debate, but one I’m sidestepping here as I’m just discussing how to track the initial emergence of these dangerous capabilities — not how to tackle them long-term.